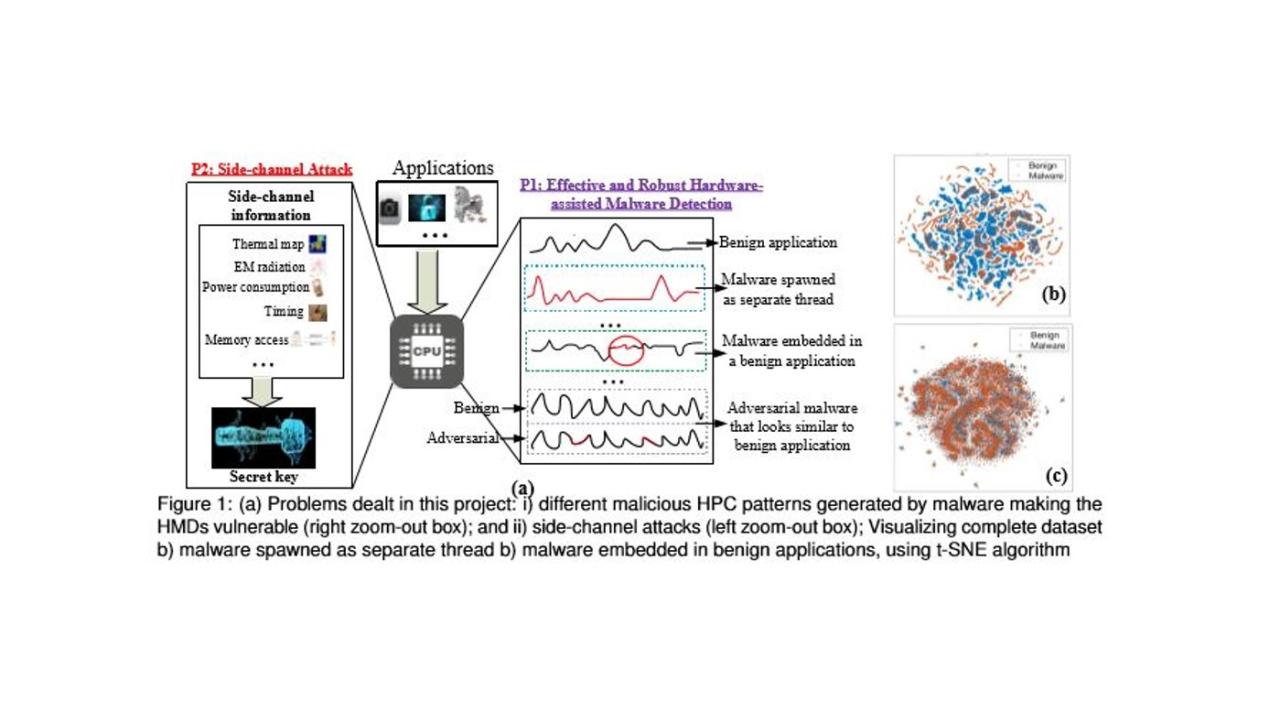
Newly Appointed Associate Professor Houman Homayoun Awarded NSF EAGER Award
Professor Houman Homayoun, a new faculty member at UC Davis ECE Department, was recently awarded the NSF EAGER award: a total of $237,134.
NSF (National Science Foundation) is a federal agency conceived by Congress in 1950. The organization supports basic scientific research, from mathematics and engineering to social sciences, and is the underpinning of funding for knowledge that transforms the future. Twenty-four percent of all federally supported basic research conducted by American universities is distributed as grants, such as the EAGER award.
The EAGER acronym stands for Early Concept Grants for Exploratory Research, awarded for particularly risky, unconventional approaches and engagement in new perspectives. “High-risk” and “high-payoff” are the two keywords attached to an EAGER award. The grant rewards scientists projects that are untested but currently in early stages of conception and its functional existence has a potentially transformative future. When asked what the most significant aspect of the project was, Prof. Homayoun responded, “being able to detect unknown malware at run-time.”
Prof. Homayoun’s proposal, titled “EAGER: Run-Time Hardware-Assisted Malware Detection Using Machine Learning”, hopes to significantly decrease malware infection through an impressive malware detection system using machine learning. Here is a short excerpt from his proposal abstract and a diagram explaining varied malware attacks that could be prevented:
“Malware, also known as malicious software is a program designed by the attackers to infect the computing systems without the user consent for serving harmful purposes such as stealing sensitive information, unauthorized data access, destroying files, running intrusive programs on devices to perform Denial-of-Service (DoS) attack, and disrupting essential services to carry out financial fraud [1, 2, 3]. Malware attacks have continued to evolve in quantity, quality and sophistication during the past decade. According to latest McAfee threat reports [4], there is nearly 37% increase in new malware in the year 2017 (more than 734 million samples in 2017). This is further exacerbated with the proliferation of mobile and Internet-of-Things (IoT). To address the latency, effectiveness and overhead shortcomings of traditional malware detection techniques such as signature-based detection [5, 6, 7, 8, 9] and semantics-based anomaly detection [10, 11, 12, 13], Hardware-assisted Malware Detection (HMD) techniques have emerged, that promises a reduced latency of malware detection process by an order of magnitude with smaller hardware cost [14, 15, 16, 17]. These HMD works differentiate normal programs by classifying anomalies using Machine Learning (ML) techniques at low-level microarchitectural feature spaces captured by Hardware Performance Counters (HPCs) [14, 16, 17, 18, 19, 20, 21] that appropriately represent the application behavior.