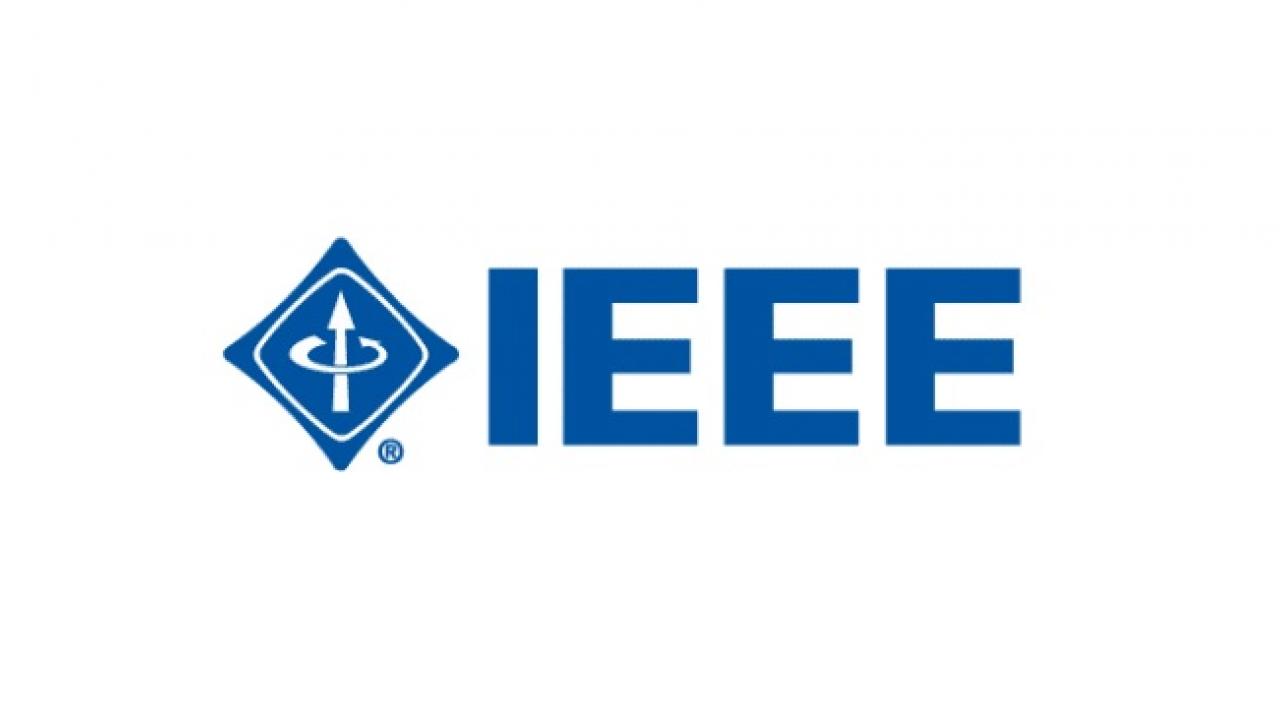
Prof. Houman Homayoun Wins Best Paper Award at 19th IEEE International Conference on Data Mining
By Adil Abbuthalha and Sumayya Shaik
Please join us in congratulating ECE’s very own Prof. Houman Homayoun and his team for best paper award at 19th IEEE ICDM (International Conference on Data Mining) in Beijing, China.
The IEEE International Conference on Data Mining (ICDM) is the internationally renowned data mining conference. Through ICDM, researchers and scholars from around the world present pioneering developments and exchange innovative experiences. “The conference covers all aspects of data mining, including algorithms, software, systems, and applications.” (wikicfp)
Out of 1046 submissions and with an acceptance rate of 9%, Prof. Houman’s paper on IoT malware confinement received the best paper award at ICDM.
The following presents a brief overview of the IoT network research done.
The sheer size of IoT networks being deployed today presents an “attack surface” and poses significant security risks at a scale never before encountered. In other words, a single device/node in a network that becomes infected with malware has the potential to spread malware across the network, eventually ceasing the network functionality.
Simply detecting and quarantining the malware in IoT networks does not guarantee to prevent malware propagation. On the other hand, use of traditional control theory for malware confinement is not effective, as most of the existing works do not consider real-time malware control strategies that can be implemented using uncertain infection information of the nodes in the network or have the containment problem decoupled from network performance.
The process of malware confinement over IoT (Internet of Things) network is typically a multi attributed graph translation problem, which is to estimate how the initial malware contact network (i.e., multi-attributed edges) and the individual IoT node (i.e., multi-attribute nodes) are jointly changed after the specific interventions. It takes the initial status of IoT network as input, and predicts the target graph which is ideally the optimal status of the network with modified connections (i.e., edges) and devices (i.e., nodes) state that helps to limit malware propagation and maintain network throughput.
Existing works on this topic are limited to either merely predicting the node attributes of graphs with fixed topology or predicting only the graph topology without considering node attributes, but cannot simultaneously predict both of them, due to substantial challenges: 1) difficulty in characterizing the interactive, iterative, and asynchronous translation process of both nodes and edges and 2) difficulty in discovering and maintaining the inherent consistency between the node and edge in predicted graphs
Since multi attributed graph translation problem is highly sophisticated, there is no generic framework yet, but only ad-hoc methods for few specific domains, which heavily rely on intensive handcrafting and domain-specific mechanistic models that could be extremely time- and resource- consuming to run on a large scale.
Hence, a generic, efficient, and end-to-end framework for general multi-attributed graph translation problems is highly in demand. In this paper Prof. Houman and his team termed this generic problem “multi attributed graph translation” and developed a novel framework integrating both node and edge translations seamlessly. The novel edge translation path is generic, which is proven to be a generalization of the existing topology translation models. Then, a spectral graph regularization based on their non-parametric graph Laplacian is proposed in order to learn and maintain the consistency of the predicted nodes and edges. Finally, extensive experiments on both synthetic and real-world application data demonstrated the effectiveness of the proposed method.
Congratulations again to Prof. Houman and his team. We are looking forward to your future accomplishments and success here at UC Davis.