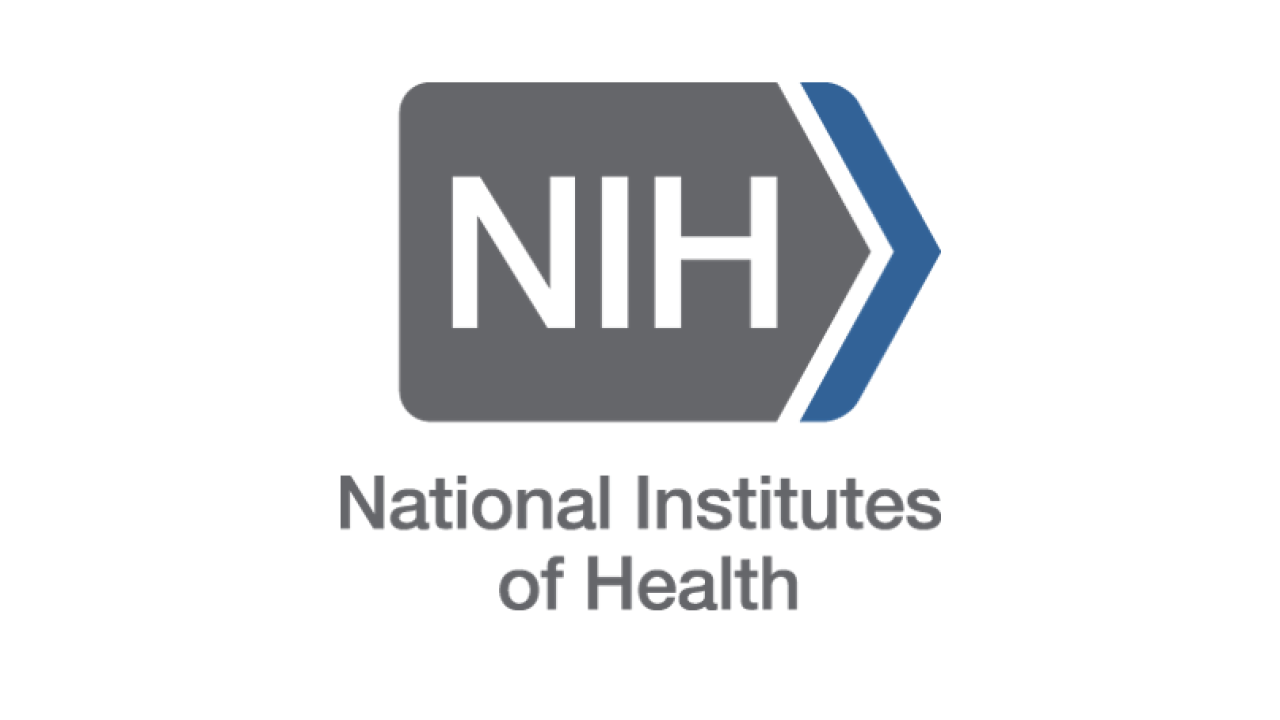
ECE Professors Will Study Autism Risk in the First Year of Life
By Adil Abbuthalha and Sumayya Shaik
Professor Chen-Nee Chuah is a co-investigator on $3.5 million NIH grant for five years to develop novel video-based approaches for detection of autism risk in the first year of life.
This project is led by Professor Sally Ozonoff from the department of Psychiatry & Behavioral Sciences in the UC Davis School of Medicine, in partnership with external co-investigator Professor Samson Cheung at University of Kentucky.
Over the last two decades, the average age of first diagnosis of autism spectrum disorder (ASD) in the United States has remained steady, at over 4 years of age, despite an average age of first parental concerns of 14 months and recent progress in understanding manifestations of ASD in infancy. Early intensive intervention has been shown to be highly promising for young children with ASD, including infants and toddlers, but is typically reserved for children with a formal diagnosis. A measure that could identify ASD risk during this period of onset offers the opportunity for intervention before the full set of symptoms is present.
PI Ozonoff and her team have developed a new video-based screening tool, the Video-referenced Infant Rating System for Autism (VIRSA), that utilizes a large library of video clips depicting a wide range of social-communication ability and relies solely on video in the ratings, with no written descriptions of behavior. They hypothesize that the semantic clarity afforded by video would provide improved early discrimination of infants at highest risk for ASD. The VIRSA was able to predict ASD diagnosis with high sensitivity across ages (6-18 months) and demonstrated 100% sensitivity (no false negatives) in concurrently identifying children showing signs of autism at 18 months of age.
Despite the demonstrated success of video-based screening, one major obstacle is the labor-intensive process of labeling and reviewing the videos manually by clinicians. In this collaborative project, we will apply computer vision and machine learning methods to videos from the VIRSA to develop a predictive model for ASD recognition. The huge video archive available for this project, with hand-coded time-stamped behavioral tags, is a highly valuable resource for machine learning. Insights gained from our exploration will pave the way for future attempts to develop video-based mobile applications for ASD recognition, which require validated classifiers that can recognize behavioral events central to early detection of ASD.